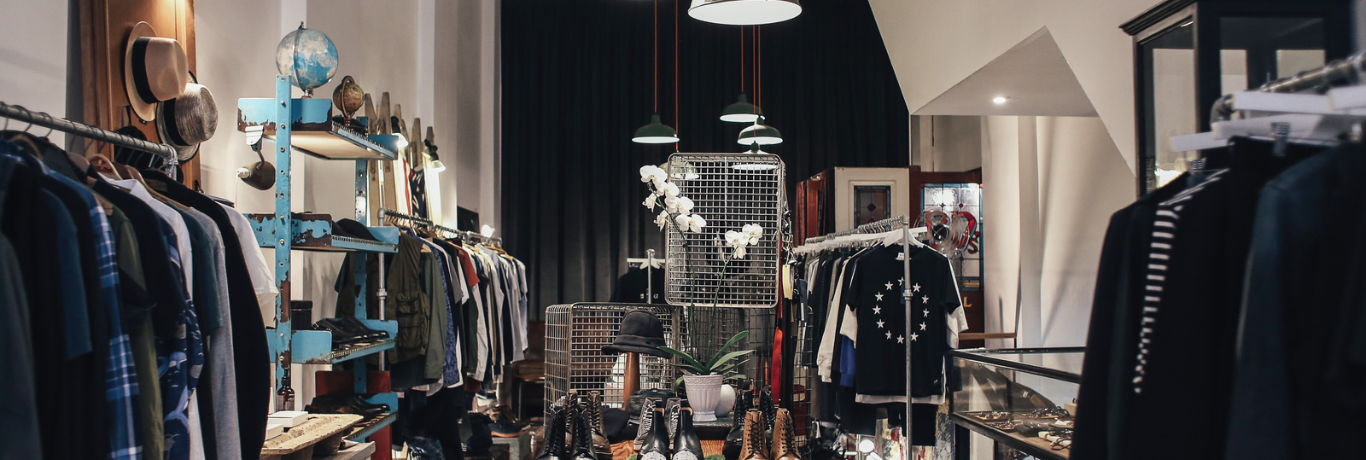
Sales Optimization - Quantifying sales shortfalls through web and in-store data analysis
Context
Our client is a leading French retailer, managing a network of hundreds of stores and generating an annual revenue of several billion euros. After a company-wide decision to place data at the heart of all departments within 2017, the client asked us to make sense of the numerous and very diverse available datasets, including: products database, sales data, websites logs, forums discussions, meteorological data. Inside this very ambitious strategic roadmap, we mainly focussed our efforts towards exploring the business of our client at its finest level: stores. The overall goal of the study was to find optimization opportunities at a local level, by exploring similarities and differences inside the network of stores.
An agile and data-driven approach to prototyping
After retrieving, cleaning and analyzing a year-long historic of the available datasets, we elaborated a list of 10 highly relevant use cases using a collaborative idea generation methodology during workshops with the client.
- Sales outliers detection
Are there products that perform particularly bad in a particular store, and why? - Products associations
What are the products that are regularly sold together? - Real-time sales analysis
How to quickly detect a product that performs badly? - Stock optimisation
How to forecast demand in order to prevent stock shortage? - Market basket analysis
What are typical basket bundles? - Forums discussions analysis
Can we detect hot topics, trends or problems mentioned inside forums discussions? - Services footprint
Which services could be relevant to offer in a given store?
What is the impact of services on sales? - Client’s projects detection
Can we detect whether a client is currently conducting a project? - Promotional impact of marketing campaigns
How advertising performs on a local level? - Sales predictions
How to forecast sales for a given store?
Very rapidly, it appeared that the relationship between in-store purchase data and website behavioral data was worth investigating to tackle a high-potential use case, particularly for retail companies: sales outliers detection.
We used a wide array of mathematical and statistical tools to detect sales outliers.
Focus on sales outliers detection prototype
The main objective of the prototype was to detect products that anomalously underperform in a particular store by measuring the gap between the estimated demand and the actual sales.
As we had the actual sales data, the tricky part was to determine the demand part. To do so, we ran 3 complementary analysis:
- Stores clustering: how a given store should perform when compared to other similar stores?
- Web sessions projection: how a given store should perform according to the website visits?
- Anomaly coefficient calculation: how does a store perform on a particular product compared to other stores?
1. Stores clustering
Store managers often compare their performance to the nearest competitors in their geographical area. However, geographical proximity does not necessarily mean similarity. To validate this reasoning, we compared sales typology across all our client’s stores and established clusters of similar stores at a nation scale.
This analysis confirmed that similarity inside a network of stores is not always driven by geography. Then, stores clusters allowed us to compare product sales among similar groups of stores and detect outliers.
Geographical proximity does not necessarily mean store similarity
2. Web sessions projection
The ROPO effect (Research Online, Purchase Offline) is today a widespread and common behavior: consumers prepare their purchase online before shopping in brick-and-mortar point of sales.
However, this behavior is pretty hard to prove and quantify, especially at a local level.
An in-depth analysis of the relationship between web logs and actual sales validated this assumption. We found that web visits were a very good proxy of sales in a particular store. This decisive analysis allowed us to predict revenue for a product by looking at web visits on a particular product, and spot items for which a stock shortage or a bad position on the shelves caused one or several missed sales.
Sales are strongly correlated to web visits for a particular product
3. Anomaly coefficient
In which of our client’s stores do certain products sell best? That is the question we answered using the anomaly coefficient method.
Adding a product-centric approach was crucial to complete the sales outliers detection analysis.
Since not all products sell the same way across different stores, we ranked the stores according to their level of sales for a particular product.
Therefore, we could quickly detect when a store was performing better or worse than the others and alert store managers in case of underperformance.
Products performance varies a lot across the network of stores
We mixed the learnings from these 3 complementary analysis in order to construct a holistic view over sales and stores performance at a national scale, allowing us to detect local discrepancies and accurately quantify sales shortfalls.
Results
Besides delivering easy-to-access optimization insights at both store and product level, one of the benefit of the sales outliers analysis was to be able to quantify its optimization potential.
While taking quite pessimistic hypothesis, we estimated that taking basic actions to fix outliers anomalies across all stores would result in a €18M additional sales on a 1-year period of time.